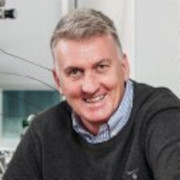
Prof Jonathan Williams: Principal investigator Organic Reactive Species research group, Max Planck Institute.
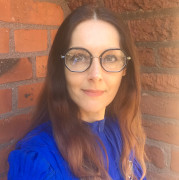
Prof Danica Kragic Jensfelt: Principal investigator Perception and Learning Lab, KTH.
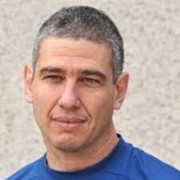
Prof Noam Sobel: Principal investigator Weizmann Olfaction Research Group; Weizmann institute of science
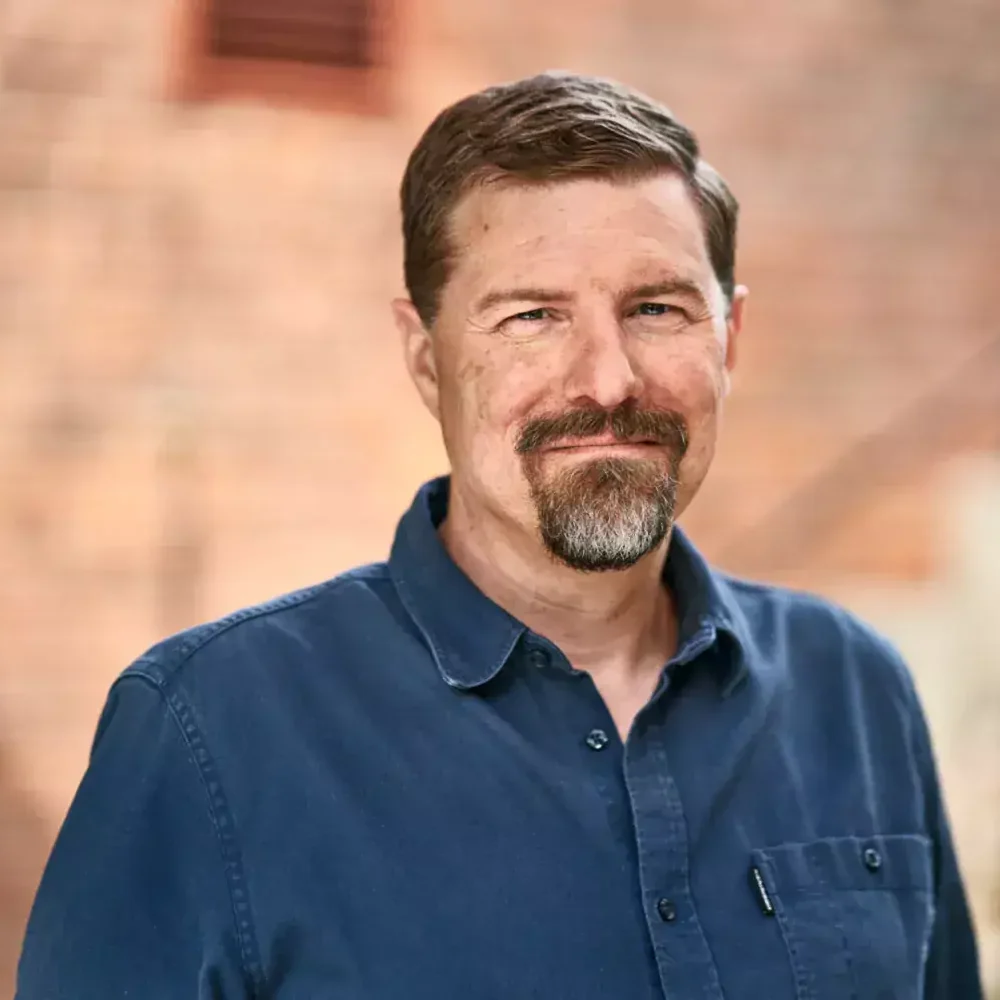
Prof Johan Lundstrom: Principal investigator Perception Neuroscience research group, Karolinska Institute.
D2Smell is an ERC-Synergy grant consortium that aims to digitize smell
We aim to digitize smell. Achieving this is currently prevented by gaps in basic science. We aim to fill these gaps, culminating in a proof of concept for our model. The primary gap we identify is lack of data on what humans typically smell. Phrased conceptually,
in Aim 1 we ask what are the natural statistics of human olfactory perceptual space. We address this in a series of three experiments, highlighted by one where we equip participants with a wearable sampling apparatus we designed and built for this proposal. The apparatus measures sniffing behaviour to identify odor sampling, measures neural activity to verify olfactory perception, takes video of the visual scene, analyses total levels of volatile organic compounds in real time, and collects odorant samples for detailed analysis off line. In other words, we generate an olfactory equivalent of Google Street View, with the addition of chemical, perceptual and neural data. Using this we will characterise the natural statistics of human olfactory perceptual space. Moreover, a major contribution of this proposal will be in posting this massive data as a publicly available recourse.
Next, in Aim 2 we build on this data to digitize human olfactory perceptual space. We put forth a model that allows us to recreate odors using a restricted set of odor primaries. We will test our model in two frameworks: One we call SmelloVision, where we develop the algorithmic framework to generate an odor to match digital images, and one we call TelleSmell, where we use a device to sense the environment, an algorithmic framework to transfer the data, and a device to generate the corresponding odor remotely. We provide pilot data for Aim 2 where we sensed an odor in Mainz (Germany), transmitted the data over IP to Rehovot (Israel), where we successfully recreated the smell. This was, as far as we know, the first transmission of odor over IP.
SMELLiT
As part of the d2smell project, we want to find out what people smell every day. Get paid for participating in research into the sense of smell. 4 times a day, at random times, the app will ask you to fill out a questionnaire on what you smell. Get a bonus for completing 40 surveys over 10 days.
Publications
All publications related to the d2smell project are shown here:
https://www.science.org/doi/10.1126/sciadv.adn3028
https://pubs.acs.org/doi/10.1021/acs.est.4c01698
Come back to see new updates!